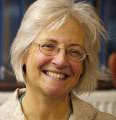
Bren Neale is Emeritus Professor of Life course and Family Research (University of Leeds, School of Sociology and Social Policy, UK) and a fellow of the Academy of Social Sciences (elected in 2010). Bren is a leading expert in Qualitative Longitudinal (QL) research methodology and provides training for new and established researchers throughout the UK and internationally.
Bren specialises in research on the dynamics of family life and inter-generational relationships, and has published widely in this field. From 2007 to 2012 she directed the Economic and Social Research Council-funded Timescapes Initiative (www.timescapes.leeds.ac.uk), as part of which she advanced QL methods across academia, and in Government and NGO settings. Since completing the ESRC funded âFollowing Young Fathers Studyâ (www.followingfathers.leeds.ac.uk) Bren has taken up a consultancy to design and support the delivery of a World Health Organisation study that is tracking the introduction of a new malaria vaccine in sub-Saharan Africa.Â
In this post, Bren draws on her extensive expertise as Director of the Timescapes Initiative, along with reflections from her forthcoming book âWhat is Qualitative Longitudinal Research?â (Bloomsbury 2018) to consider the diverse forms of archival data that may be re-used or re-purposed in qualitative longitudinal work. In so doing, Bren outlines the possibilities for, and progress made in developing ways of working with and across assemblages of archived materials to capture social and temporal processes.
 Research Data as Documents of Life
Among the varied sources of data that underpin Qualitative Longitudinal (QL) studies, documentary and archival sources have been relatively neglected. This is despite their potential to shed valuable light on temporal processes. These data sources form part of a larger corpus of materials that Plummer (2001) engagingly describes as âdocuments of lifeâ:
âThe world is crammed full of human personal documents. People keep diaries, send letters, make quilts, take photos, dash off memos, compose auto/biographies, construct websites, scrawl graffiti, publish their memoirs, write letters, compose CVs, leave suicide notes, film video diaries, inscribe memorials on tombstones, shoot films, paint pictures, make tapes and try to record their personal dreams. All of these expressions of personal life are hurled out into the world by the millions, and can be of interest to anyone who cares to seek them outâ (p. 17).
To take one example, letters have long provided a rich source of insight into unfolding lives. In their classic study of Polish migration, conducted in the first decades of the twentieth century, Thomas and Znaniecki (1958 [1918-20)] analysed the letters of Polish migrants to the US (an opportunistic source, for a rich collection of such letters was thrown out of a Chicago window and landed at Znanieckiâs feet). Similarly, Stanleyâs (2013) study of the history of race and apartheid was based on an analysis of three collections of letters written by white South Africans spanning a 200 year period (1770s to 1970s). The documentary treasure trove outlined by Plummer also includes articles in popular books, magazines and newsprint; text messages, emails and interactive websites; the rich holdings of public record offices; and confidential and often revealing documents held in organisations and institutions. Social biographers and oral historians are adept at teasing out a variety of such evidence to piece together a composite picture of lives and times; they are âjackdawsâ rather than methodological purists (Thompson 1981: 290).
Among the many forms of documentary data that may be repurposed by researchers, social science and humanities datasets have significant value. The growth in the use of such legacy data over recent decades has been fuelled by the enthusiasm and commitment of researchers who wish to preserve their datasets for historical use. Further impetus has come from the development of data infrastructures and funding initiatives to support this process, and a fledgling corpus of literature that is documenting and refining methodologies for re-use (e.g. Corti, Witzel and Bishop 2005; Crow and Edwards 2012; Irwin 2013). Alongside the potential to draw on individual datasets, there is a growing interest in working across datasets, bringing together data that can build new insights across varied social or historical contexts (e.g. Irwin, Bornat and Winterton 2012; and indeed the project on which this website is founded).
Many qualitative datasets remain in the stewardship of the original researchers where they are at risk of being lost to posterity (although they may be fortuitously rediscovered, OâConnor and Goodwin 2012). However, the culture of archiving and preserving legacy data through institutional, specialist or national repositories is fast becoming established (Bishop and Kuula-Luumi 2017). These facilities are scattered across the UK (for example, the Kirklees Sound Archive in West Yorkshire, which houses oral history interviews on the wool textile industry (Bornat 2013)). The principal collections in the UK are held at the UK Data Archive (which includes the classic âQualidataâ collection); the British Library Sound Archive, NIQA (the Northern Ireland Qualitative Archive, including the ARK resource); the recently developed Timescapes Archive (an institutional repository at the University of Leeds, which specialises in Qualitative Longitudinal datasets); and the Mass Observation Archive, a resource which, for many decades, has commissioned and curated contemporary accounts from a panel of volunteer recorders. International resources include the Irish Qualitative Data Archive, the Murray Research Center Archive (Harvard), and a range of data facilities at varying levels of development across mainland Europe (Neale and Bishop 2010-11).
In recent years some vigorous debates have ensued about the ethical and epistemological foundations for reusing qualitative datasets. In the main, the issues have revolved around data ownership and researcher reputations; the ethics of confidentiality and consent for longer-term use; the nature of disciplinary boundaries; and the tension between realist understandings of data (as something that is simply âout thereâ), and a narrowly constructivist view that data are non-transferable because they are jointly produced and their meaning tied to the context of their production.
These debates are becoming less polarised over time. In part this is due to a growing awareness that most of these issues are not unique to the secondary use of datasets (or documentary sources more generally) but impact also on their primary use, and indeed how they are generated in the first place. In particular, epistemological debates about the status and veracity of qualitative research data are beginning to shift ground (see, for example, Mauthner et al 1998 and Mauthner and Parry 2013). Research data are by no means simply âout thereâ for they are inevitably constructed and re-constructed in different social, spatial and historical contexts; indeed, they are transformed historically simply through the passage of time (Moore 2007). But this does not mean that the narratives they contain are âmade upâ or that they have no integrity or value across different contexts (Hammersley 2010; Bornat 2013). It does suggest, however, that data sources are capable of more than one interpretation, and that their meaning and salience emerge in the moment of their use:
âThere is no a-priori privileged moment in time in which we can gain a deeper, more profound, truer insight, than in any other moment. ⌠There is never a single authorised reading ⌠It is the multiple viewpoints, taken together, which are the most illuminatingâ (Brockmeier and Reissman cited in Andrews 2008: 89; Andrews 2008: 90).
Moreover, whether revisiting data involves stepping into the shoes of an earlier self, or of someone else entirely, this seems to have little bearing on the interpretive process. From this point of view, the distinctions between using and re-using data, or between primary and secondary analysis begin to break down (Moore 2007; Neale 2013).
This is nowhere more apparent than in Qualitative Longitudinal enquiry, where the transformative potential of data is part and parcel of the enterprise. Since data are used and re-used over the longitudinal frame of a study, their re-generation is a continual process. The production of new data as a study progresses inevitably reconfigures and re-contextualises the dataset as a whole, creating new assemblages of data and opening up new insights from a different temporal standpoint. Indeed, since longitudinal datasets may well outlive their original research questions, it is inevitable that researchers will need to ask new questions of old data (Elder and Taylor 2009).
The status and veracity of research data, then, is not a black and white, either/or issue, but one of recognising the limitations and partial vision of all data sources, and the need to appraise the degree of âfitâ and contextual understanding that can be achieved and maintained (Hammersley 2010; Duncan 2012; Irwin 2013). This, in turn, has implications for how a dataset is crafted and contextualised for future use (Neale 2013).
A decade ago, debates about the use of qualitative datasets were in danger of becoming polarised (Moore 2007). However, the pre-occupations of researchers are beginning to move on. The concern with whether or not qualitative datasets should be used is giving way to a more productive concern with how they should be used, not least, how best to work with their inherent temporality. Overall, the âjackdawâ approach to re-purposing documentary and archival sources of data is the very stuff of historical sociology and of social history more generally (Kynaston 2005; Bornat 2008; McLeod and Thomson 2009), and it has huge and perhaps untapped potential in Qualitative Longitudinal research.
References
Andrews, M. (2008) âNever the last word: Revisiting dataâ, in M. Andrews, C. Squire and M. Tamboukou (eds.) Doing narrative research, London, Sage, 86-101
Bishop, L. and Kuula-Luumi, A. (2017) âRevisiting Qualitative Data reuse: A decade onâ, SAGE Open, Jan-March, 1-15.
Bornat, J. (2008) Crossing boundaries with secondary analysis: Implications for archived oral history data, Paper given at the ESRC National Council for Research Methods Network for Methodological Innovation: Theory, Methods and Ethics across Disciplines, 19 September 2008, University of Essex,
Bornat, J. (2013) âSecondary analysis in reflection: Some experiences of re-use from an oral history perspectiveâ, Families, Relationships and Societies, 2, 2, 309-17
Corti, L., Witzel, A. and Bishop, L. (2005) (eds.) Secondary analysis of qualitative data: Special issue, Forum: Qualitative Social Research, 6,1.
Crow, G. and Edwards, R. (2012) (eds.) â Editorial Introduction: Perspectives on working with archived textual and visual material in social researchâ, International Journal of Social Research Methodology, 15,4, 259-262.
Duncan, S. (2012) âUsing elderly data theoretically: Personal life in 1949/50 and individualisation theoryâ, International Journal of Social Research Methodology, 15, 4, 311-319.
Elder, G. and Taylor, M. (2009) âLinking research questions to data archivesâ, in J. Giele and G. Elder (eds.) The Craft of Life course research, New York, Guilford Press, Â 93-116.
Hammersley, M. (2010) âCan we re-use qualitative data via secondary analysis? Notes on some terminological and substantive issuesâ, Sociological Research Online, 15, 1,5.
Irwin, S. (2013) âQualitative secondary analysis in practiceâ Introductionâ in S. Irwin and J. Bornat (eds.) Qualitative secondary analysis (Open Space), Families, Relationships and Societies, 2, 2, 285-8.
Irwin, S., Bornat, J. and Winterton, M. (2012) âTimescapes secondary analysis: Comparison, context and working across datasetsâ, Qualitative Research, 12, 1, 66-80
Kynaston, D. (2005) âThe uses of sociology for real-time history,â Forum: Qualitative Social Research, 6,1.
McLeod, J. and Thomson, R. (2009) Researching Social Change: Qualitative approaches, Sage.
Mauthner, N., Parry, O. and Backett-Milburn, K. (1998) âThe data are out there, or are they? Implications for archiving and revisiting qualitative dataâ, Sociology, 32,4, 733-745.
Mauthner, N. and Parry, O. (2013). âOpen Access Digital Data Sharing: Principles, policies and practicesâ, Social Epistemology, 27, 1, 47-67.
Moore, N. (2007) â(Re) using qualitative data?â Sociological Research Online, 12, 3, 1.
Neale, B. (2013)â Adding time into the mix: Stakeholder ethics in qualitative longitudinal researchâ, Methodological Innovations Online, 8, 2, 6-20.
Neale, B., and Bishop, L. (2010 -11) âQualitative and qualitative longitudinal resources in Europe: Mapping the fieldâ, IASSIST Quarterly: Special double issue, 34 (3-4); 35(1-2)
OâConnor, H. and Goodwin, J. (2012) âRevisiting Norbert Eliasâs sociology of community: Learning from the Leicester re-studiesâ, The Sociological Review, 60, 476-497.
Plummer, K. (2001) Documents of Life 2: An invitation to a critical humanism, London, Sage.
Stanley, L. (2013) âWhites writing: Letters and documents of life in a QLR projectâ, in L. Stanley (ed.) Documents of life revisited, London, Routledge, 59-76.
Thomas, W. I. and Znaniecki, F. (1958) [1918-20] The Polish Peasant in Europe and America Volumes I and II, New York, Dover Publications.
Thompson, P. (1981) âLife histories and the analysis of social changeâ, in. D. Bertaux (ed.) Biography and society: the life history approach in the social sciences, London, Sage, 289-306.