In todayâs blog, Dr Elena Zaitseva, an Academic Research and Development Officer at the Teaching and Learning Academy, Liverpool John Moores University, describes her search for a user-friendly instrument that enables researchers to get an overview of an entire data landscape. She uses the text analytics tool Leximancer to conduct automated semantic analysis of responses to open questions in surveys; data that often goes unanalysed.
Elenaâs research interests are in the higher education student experience, learner identity and learner journeys.  She has been using the semantic analysis software Leximancer for analysis of large qualitative data sets since 2011. Outcomes of this research are published in the Quality in Higher Education Journal, several book chapters and in two reports commissioned by the Higher Education Academy (now Advance HE).
Navigating the landscape of qualitative data in surveys with automated semantic analysis
Reflecting on the quantitative-qualitative divide in large scale survey data almost twenty years ago, Â Bolden and Moscarola (2000) concluded that free text comments (e.g. responses to open questions in questionnaires) are âpoorly utilised, either being totally ignored, analysed non-systematically, or treated as an asideâ (Bolden and Moscarola, 2000, p. 450). Two decades later and not much has changed. Â Examining thousands of fragmented open question responses, varying from a short phrase or sentence to mini-narratives or lengthy reflective accounts, remains a complex, time and resource consuming exercise for researchers. However, timely analysis of free text comments could help not only enhance understanding of quantitative results, but also reveal new discourses not necessarily anticipated by the surveyâs creators.
As part of a Higher Education Funding Council for England (HEFCE) funded project on the âSophomore Slumpâ that investigated disengagement and underperformance of second year university students, we undertook a comparative analysis of comments provided in a student survey deployed at each level of study (comparing themes from year one, two and final year studentsâ feedback) (Zaitseva et al, 2013). Each data set comprised, on average, 250 pages of text – single spaced Times New Roman 12 point font.
My search for a user-friendly instrument that would allow us to instantly see the whole institutional landscape of student feedback for each level of study, and be able to detect differences and drill down into the particular areas or topics, led me to Leximancer â a tool for visualising the conceptual and thematic structure of a text, developed at the University of Queensland (Smith and Humphreys, 2006).
The software automatically identifies concepts, themes (clusters of concepts) and connections between them by data mining the text, and visually representing the findings in the form of a concept map â a process called unsupervised semantic mapping of natural language. Based on an assumption that a concept is characterised by words that tend to appear in conjunction with it, the software measures how relevant one word is to a set of other words. Only words that pass a certain relevance weight threshold, established by the software, form concepts, although this parameter can be manually adjusted (Fig 1).
Figure 1. Example of a concept map generated by Leximancer
The tool not only determines the key concepts, themes and associated sentiments, but also provides useful information about the proximity of the concepts and their location. This is particularly beneficial for longitudinal and comparative analysis where underlying differences can be identified from the positioning of concepts on the map.
Although the âmappingâ process is completed automatically, making sense of the map and establishing meaning behind each concept is the researcherâs task. The researcher has to âdissectâ the concepts and associated themes by exploring all instances (direct quotes) that contributed to the conceptâs creation, and undertake a more traditional interpretive/thematic analysis.
Using Leximancer in the âSophomore Slumpâ research helped uncover change in student attitudes and priorities as they progressed with their studies, showing how they moved from affectively oriented goals in the first year to a second yearâs learning and goal reaffirmation stage, and achievement and outcome-oriented learning in the final year.
Another research project where the capabilities of Leximancer were tested, was analysis of free text comments of postgraduate taught students at the sector level to identify the dominant themes within their feedback (Zaitseva and Milsom, 2015). The Postgraduate Taught Experience Survey (PTES) dataset included responses of 67,580 students from 100 higher education institutions. The survey provided the opportunity to comment after each section (seven in total), and invited responses on the most enjoyable aspects as well as how the course experience could be improved. The overall data set comprised around 2,670,000 words which was the equivalent of 5933 pages (single spaced Times New Roman, 12 point font). An attempt to generate a concept map from a combined data set resulted in a densely populated map and thousands of quotes attached to each concept, so it was deemed unsuitable for analysis. The data had to be disaggregated by analysing responses from each section separately, and augmented by insights from the demographic data breakdown (e.g. looking at trends in responses of young and mature, part-time and full-time students) to be able to achieve at least some saturation in thematic exploration.
The analysis identified a number of new themes, including the heavy workload of part-time students which was often underrepresented in course-related marketing information, and its impact on student mental health and ability to achieve (Fig 2.); issues around âlevelnessâ of Masters programme delivery which, in some cases, was aimed at doctoral level and, in other cases, at final year undergraduate degree, and some other.
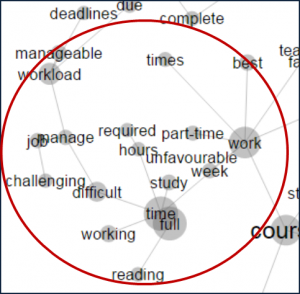
Figure 2. A fragment of part-time student experience concept map
Instruments such as Leximancer allow researchers to conduct analysis of large qualitative data sets in a time-efficient and consistent manner, as data pre-processing is done by computer. The concept map that emerges from this analysis captures âthe wisdom of crowdsâ (Dodgson et al. 2008) and is a text-driven, not a researcher-driven representation. But the researcher is able to interrogate the concept map and perform a more focused/tailored analysis by mining the text for âdeeper contextual associationsâ (Stewart and Chakraborty, 2010). The vaster the data source, the more nuanced the concept map will be.
Use of computer aided analysis increases reliability (as the top level of analysis is independent of the researcherâs effect), and facilitates reproducibility of the findings as it is possible to retrace your thinking that may have contributed to the emergence of new ideas and research findings.
There are limitations to this type of analysis. Some concepts emerge strongly where they are represented by a narrow vocabulary. In the context of student surveys, words such as lecture, library, feedback or exams will have a strong presence on the concept maps. In contrast, other elements of student experience, such as personal development or extracurricular activities, will be identified from a broader pool of terms and have a greater likelihood of being diluted as a concept in the map. This can be mitigated by undertaking a tailored analysis, for example, through concept seeding, by adding concepts that have not passed publication threshold, but are of interest to the researcher.
Some concepts are relatively fixed in their meaning, while others are very broad. For instance, the concept tutorial is most likely to represent a single meaning in student feedback. At the same time, the concept work, being noun as well as a verb, might have multiple meanings. To fine-tune the analysis, more specific queries should be run to better understand all connotations related to the concept (e.g. group + work, part-time+ work).
Sentiment analysis needs to be occasionally verified by checking contextual understanding, but Leximancer also mitigates this by providing both indicators (favourable and unfavourable probability).
Without any doubt there are limits to what the software analysis can achieve. Complexity of language implies that automated semantic analysis methods will never replace careful and close reading of the text, but âcomputer assisted methods are best thought of as amplifying and augmenting careful reading and thoughtful analysisâ (Grimmer and Stewart, 2013, p. 2). These methods are vital to handling large volumes of qualitative data that might otherwise go un-analysed.
References
Bolden, R. and Moscarola, J. (2000) Bridging the Quantitative-Qualitative Divide: The Lexical Approach to Textual Data Analysis, Social Science Computer Review, 18(4): 450-460.
Grimmer, J. and Stewart, B. (2013) Text as Data: The Promise and Pitfalls of Automatic Content Analysis Methods for Political Texts, Political Analysis Advance Access, 1-31, available online:Â https://web.stanford.edu/~jgrimmer/tad2.pdf
Smith, A. and Humpreys, M. (2006) Evaluation of Unsupervised Semantic Mapping of Natural Language with Leximancer Concept Mapping, Behavioural Research Methods, (38): 262â79
Stewart, G. and Chakraborty, A. (2010) Strategy Content Analysis for Service Identification: A Case Study on Government Agencies. 5th Conference on Qualitative Research in IT, Brisbane, available online:Â https://researchonline.jcu.edu.au/25633/1/QUALIT10.pdf
Zaitseva, E., Milsom, C. and Stewart, M. (2013) Connecting the Dots: Using Concept Maps for Interpreting Student Satisfaction. Quality in Higher Education, 19(2): 225â47.
Zaitseva, E. and Milsom, C. (2015) In their own words: Analysing studentsâ comments from the Postgraduate Taught Experience Survey, York: Higher Education Academy, available online: https://www.heacademy.ac.uk/knowledge-hub/postgraduate-taught-experience-survey-2015
Dec 11
Guest post#19: Dr Elena Zaitseva: Navigating the landscape of qualitative data in surveys with automated semantic analysis
Elenaâs research interests are in the higher education student experience, learner identity and learner journeys.  She has been using the semantic analysis software Leximancer for analysis of large qualitative data sets since 2011. Outcomes of this research are published in the Quality in Higher Education Journal, several book chapters and in two reports commissioned by the Higher Education Academy (now Advance HE).
Navigating the landscape of qualitative data in surveys with automated semantic analysis
Reflecting on the quantitative-qualitative divide in large scale survey data almost twenty years ago, Â Bolden and Moscarola (2000) concluded that free text comments (e.g. responses to open questions in questionnaires) are âpoorly utilised, either being totally ignored, analysed non-systematically, or treated as an asideâ (Bolden and Moscarola, 2000, p. 450). Two decades later and not much has changed. Â Examining thousands of fragmented open question responses, varying from a short phrase or sentence to mini-narratives or lengthy reflective accounts, remains a complex, time and resource consuming exercise for researchers. However, timely analysis of free text comments could help not only enhance understanding of quantitative results, but also reveal new discourses not necessarily anticipated by the surveyâs creators.
As part of a Higher Education Funding Council for England (HEFCE) funded project on the âSophomore Slumpâ that investigated disengagement and underperformance of second year university students, we undertook a comparative analysis of comments provided in a student survey deployed at each level of study (comparing themes from year one, two and final year studentsâ feedback) (Zaitseva et al, 2013). Each data set comprised, on average, 250 pages of text – single spaced Times New Roman 12 point font.
My search for a user-friendly instrument that would allow us to instantly see the whole institutional landscape of student feedback for each level of study, and be able to detect differences and drill down into the particular areas or topics, led me to Leximancer â a tool for visualising the conceptual and thematic structure of a text, developed at the University of Queensland (Smith and Humphreys, 2006).
The software automatically identifies concepts, themes (clusters of concepts) and connections between them by data mining the text, and visually representing the findings in the form of a concept map â a process called unsupervised semantic mapping of natural language. Based on an assumption that a concept is characterised by words that tend to appear in conjunction with it, the software measures how relevant one word is to a set of other words. Only words that pass a certain relevance weight threshold, established by the software, form concepts, although this parameter can be manually adjusted (Fig 1).
The tool not only determines the key concepts, themes and associated sentiments, but also provides useful information about the proximity of the concepts and their location. This is particularly beneficial for longitudinal and comparative analysis where underlying differences can be identified from the positioning of concepts on the map.
Although the âmappingâ process is completed automatically, making sense of the map and establishing meaning behind each concept is the researcherâs task. The researcher has to âdissectâ the concepts and associated themes by exploring all instances (direct quotes) that contributed to the conceptâs creation, and undertake a more traditional interpretive/thematic analysis.
Using Leximancer in the âSophomore Slumpâ research helped uncover change in student attitudes and priorities as they progressed with their studies, showing how they moved from affectively oriented goals in the first year to a second yearâs learning and goal reaffirmation stage, and achievement and outcome-oriented learning in the final year.
Another research project where the capabilities of Leximancer were tested, was analysis of free text comments of postgraduate taught students at the sector level to identify the dominant themes within their feedback (Zaitseva and Milsom, 2015). The Postgraduate Taught Experience Survey (PTES) dataset included responses of 67,580 students from 100 higher education institutions. The survey provided the opportunity to comment after each section (seven in total), and invited responses on the most enjoyable aspects as well as how the course experience could be improved. The overall data set comprised around 2,670,000 words which was the equivalent of 5933 pages (single spaced Times New Roman, 12 point font). An attempt to generate a concept map from a combined data set resulted in a densely populated map and thousands of quotes attached to each concept, so it was deemed unsuitable for analysis. The data had to be disaggregated by analysing responses from each section separately, and augmented by insights from the demographic data breakdown (e.g. looking at trends in responses of young and mature, part-time and full-time students) to be able to achieve at least some saturation in thematic exploration.
The analysis identified a number of new themes, including the heavy workload of part-time students which was often underrepresented in course-related marketing information, and its impact on student mental health and ability to achieve (Fig 2.); issues around âlevelnessâ of Masters programme delivery which, in some cases, was aimed at doctoral level and, in other cases, at final year undergraduate degree, and some other.
Figure 2. A fragment of part-time student experience concept map
Instruments such as Leximancer allow researchers to conduct analysis of large qualitative data sets in a time-efficient and consistent manner, as data pre-processing is done by computer. The concept map that emerges from this analysis captures âthe wisdom of crowdsâ (Dodgson et al. 2008) and is a text-driven, not a researcher-driven representation. But the researcher is able to interrogate the concept map and perform a more focused/tailored analysis by mining the text for âdeeper contextual associationsâ (Stewart and Chakraborty, 2010). The vaster the data source, the more nuanced the concept map will be.
Use of computer aided analysis increases reliability (as the top level of analysis is independent of the researcherâs effect), and facilitates reproducibility of the findings as it is possible to retrace your thinking that may have contributed to the emergence of new ideas and research findings.
There are limitations to this type of analysis. Some concepts emerge strongly where they are represented by a narrow vocabulary. In the context of student surveys, words such as lecture, library, feedback or exams will have a strong presence on the concept maps. In contrast, other elements of student experience, such as personal development or extracurricular activities, will be identified from a broader pool of terms and have a greater likelihood of being diluted as a concept in the map. This can be mitigated by undertaking a tailored analysis, for example, through concept seeding, by adding concepts that have not passed publication threshold, but are of interest to the researcher.
Some concepts are relatively fixed in their meaning, while others are very broad. For instance, the concept tutorial is most likely to represent a single meaning in student feedback. At the same time, the concept work, being noun as well as a verb, might have multiple meanings. To fine-tune the analysis, more specific queries should be run to better understand all connotations related to the concept (e.g. group + work, part-time+ work).
Sentiment analysis needs to be occasionally verified by checking contextual understanding, but Leximancer also mitigates this by providing both indicators (favourable and unfavourable probability).
Without any doubt there are limits to what the software analysis can achieve. Complexity of language implies that automated semantic analysis methods will never replace careful and close reading of the text, but âcomputer assisted methods are best thought of as amplifying and augmenting careful reading and thoughtful analysisâ (Grimmer and Stewart, 2013, p. 2). These methods are vital to handling large volumes of qualitative data that might otherwise go un-analysed.
References
Bolden, R. and Moscarola, J. (2000) Bridging the Quantitative-Qualitative Divide: The Lexical Approach to Textual Data Analysis, Social Science Computer Review, 18(4): 450-460.
Grimmer, J. and Stewart, B. (2013) Text as Data: The Promise and Pitfalls of Automatic Content Analysis Methods for Political Texts, Political Analysis Advance Access, 1-31, available online:Â https://web.stanford.edu/~jgrimmer/tad2.pdf
Smith, A. and Humpreys, M. (2006) Evaluation of Unsupervised Semantic Mapping of Natural Language with Leximancer Concept Mapping, Behavioural Research Methods, (38): 262â79
Stewart, G. and Chakraborty, A. (2010) Strategy Content Analysis for Service Identification: A Case Study on Government Agencies. 5th Conference on Qualitative Research in IT, Brisbane, available online:Â https://researchonline.jcu.edu.au/25633/1/QUALIT10.pdf
Zaitseva, E., Milsom, C. and Stewart, M. (2013) Connecting the Dots: Using Concept Maps for Interpreting Student Satisfaction. Quality in Higher Education, 19(2): 225â47.
Zaitseva, E. and Milsom, C. (2015) In their own words: Analysing studentsâ comments from the Postgraduate Taught Experience Survey, York: Higher Education Academy, available online: https://www.heacademy.ac.uk/knowledge-hub/postgraduate-taught-experience-survey-2015